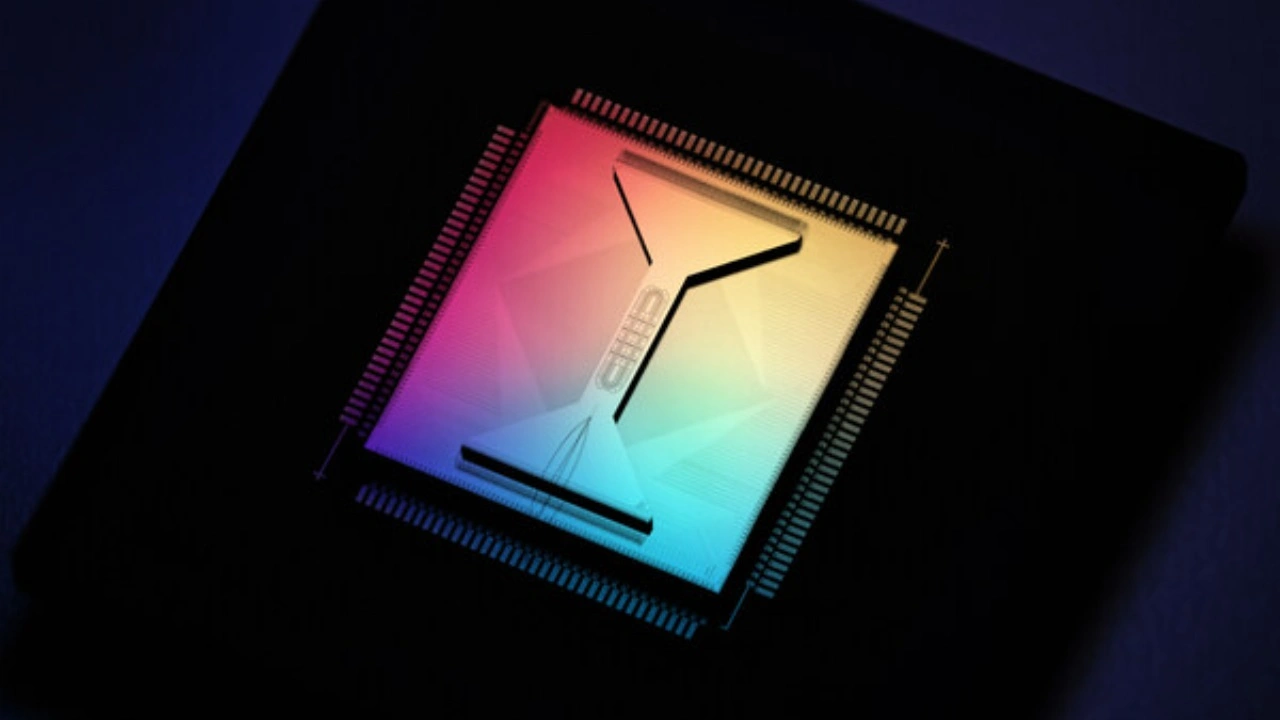
Recent studies have revealed that quantum artificial intelligence can help decipher how large language models, such as those used in chatbots like ChatGPT, generate their responses. Interpretability is a crucial aspect of responsible AI, yet many systems function as “black boxes,” making it challenging for users to understand the sources of errors and how to rectify them.
Researchers at Quantinuum have published findings demonstrating their innovative integration of quantum computing with artificial intelligence, specifically in the realm of text-level quantum natural language processing (QNLP). They introduced a new model named QDisCoCirc, which successfully illustrates how to train a quantum computer model in a way that is both interpretable and scalable for text-based applications.
The team focused on achieving “compositional interpretability,” which allows for human-readable meanings to be assigned to the model’s components, thereby clarifying how these parts work together. This transparency is vital in fields such as healthcare, finance, pharmaceuticals, and cybersecurity, where AI systems are increasingly subject to regulatory scrutiny to ensure ethical behavior and comprehensible outputs.
Quantinuum enhanced the scalability of their approach by employing “compositional generalization,” allowing for small-scale training on classical computers before testing more complex examples that traditional computers cannot simulate on quantum systems.
The researchers explained, “The compositional structure enables us to examine and interpret the word embeddings that the model learns for each term, as well as the interactions between them. This enhances our understanding of how it approaches question-answering tasks.”
This compositional strategy also addresses the trainability challenges linked to the “barren plateau” phenomenon commonly encountered in conventional quantum machine learning (QML). This issue occurs when the gradient indicating the model’s predictive accuracy flattens out as the system scales, making it harder to identify pathways for improvement.
The new methodology increases the efficiency and scalability of large quantum models for intricate tasks. The team demonstrated this capability using Quantinuum’s H1-1 trapped-ion quantum processor, marking the first proof of concept for scalable compositional QNLP.
Ilyas Khan, founder and chief product officer of Quantinuum, noted, “Earlier this summer, we released a comprehensive technical paper outlining our commitment to responsible and safe AI—systems that are inherently transparent and secure. This research foreshadowed experimental work demonstrating how this can operate at scale on quantum computers. I am excited to share this next development, which includes extensive details now publicly available. Natural language processing is fundamental to large language models, and our approach continues to enhance our ambitious efforts across various applications, from chemistry to cybersecurity and AI.”