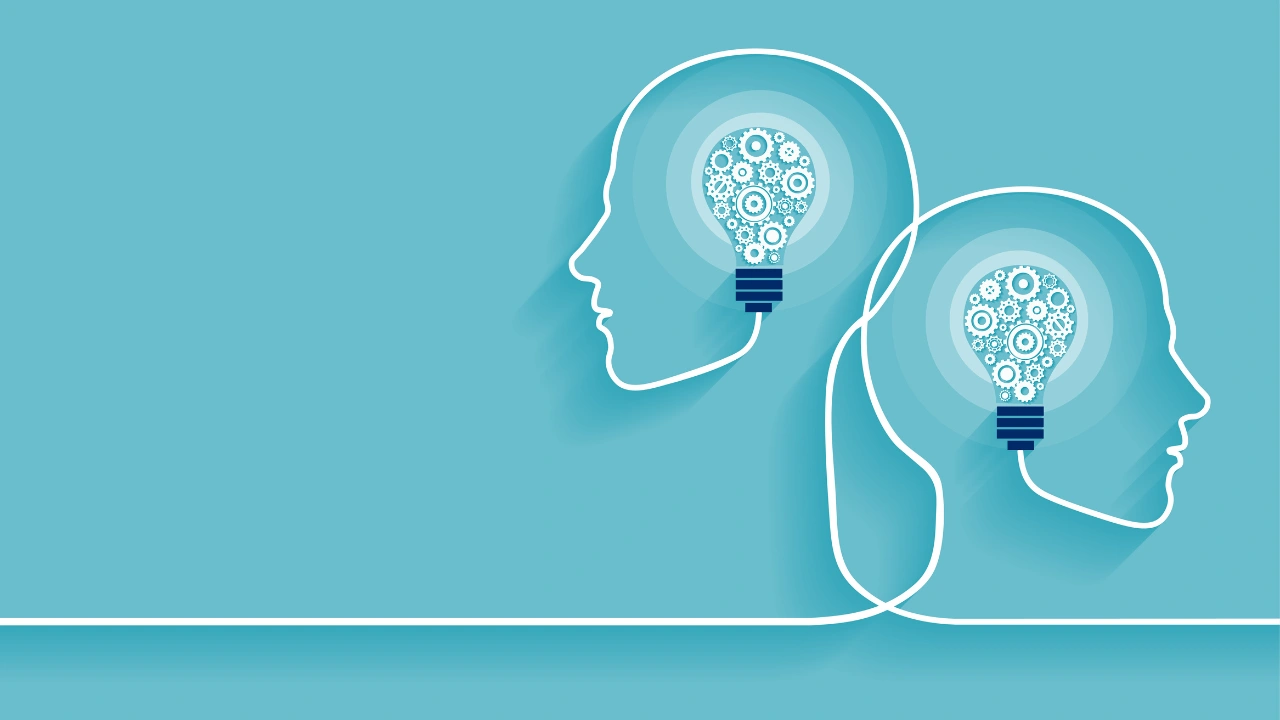
A team of robotics researchers from UC Berkeley, Stanford University, and the University of Warsaw has introduced a groundbreaking approach to improve robotic interaction with their environments. This new method, known as Embodied Chain-of-Thought Reasoning (ECoT), equips robots with the ability to methodically process tasks and assess their surroundings before taking action.
In a recently published paper, the researchers explain that ECoT aims to enhance a robot’s capability to tackle unfamiliar tasks and navigate various environments more efficiently. Additionally, it offers human operators a means to adjust a robot’s decision-making through natural language feedback.
Vision-language-action models (VLAs) have emerged as effective tools for training robots in task execution. These models help robots gain a better understanding of assigned tasks, as noted by researchers from Google DeepMind in a June 2023 study.
However, the researchers pointed out that traditional VLAs often rely on observational learning without integrating intermediate reasoning. This limitation hinders their ability to manage complex and novel situations that necessitate thoughtful planning and adaptability.
To overcome these challenges, the research team incorporated a foundation model into their work. They created a scalable framework for generating synthetic training data for ECoT by utilizing various foundation models to extract features from robot demonstrations found in the Bridge V2 dataset.
By employing a collection of foundation models, including object detectors and vision-language models, the team was able to produce detailed descriptions of the robot’s environment, including annotations for identified objects.
They then utilized Google’s Gemini model to devise plans, subtasks, and movement labels, merging this data with the previously collected information about the scene and the robot’s gripper position. This modular approach allowed the robots to engage in a thorough reasoning process before executing their tasks.
Notably, the researchers discovered that the ECoT reasoning framework could be adapted to different robot embodiments, enabling the policy to generalize its reasoning abilities across robots not included in the training phase.
The implementation of ECoT led to a significant improvement in the success rate of OpenVLA, an open-source VLA, achieving a 28% increase in performance on challenging generalization tasks without the need for additional robot training data.
Despite its advantages, the method has certain limitations. The fixed sequence of reasoning steps could restrict the robot’s adaptability in rapidly changing environments. The researchers acknowledged that enhancing the project with a larger dataset would allow ECoT to be applied to a wider range of robots.
Additionally, they are exploring ways to optimize control frequencies to improve execution speed, as this remains a potential bottleneck in the process.
The interest in foundation models continues to grow within the robotics field, with implications for enabling robots to perform more generalized tasks. A startup named Skild AI is aiming to leverage this research to reduce the costs associated with robotics training. Recently, Skild secured $300 million in funding, with its foundation model already being utilized for automation solutions in visual inspection and patrolling tasks.